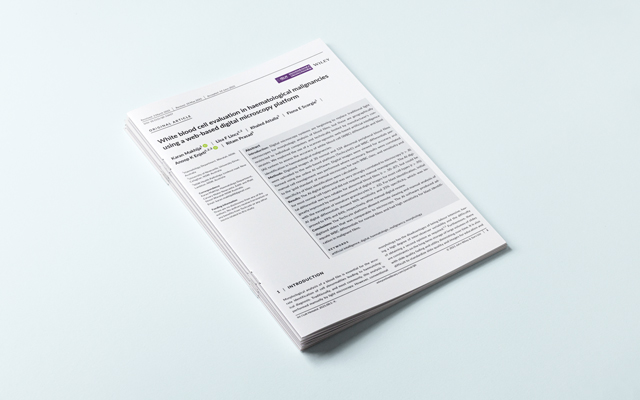
—- the following is taken from the International Society for Laboratory Hematology paper that can be viewed here. —-
Abstract
Introduction: Digital microscopy systems are beginning to replace traditional light microscopes for morphologic analysis of blood films, but these are geographically restricted to individual computers and technically limited by manufacturer’s constraints. We explored the use of a scanner-agnostic web-based artificial intelligence (AI) system to assess the accuracy of white blood cell (WBC) differentials and blast identification in haematological malignancies.
Methods: Digitized images of 20 normal and 124 abnormal peripheral blood films were uploaded to the web-based platform (Techcyte©) and WBC differentials performed using the online AI software. Digital images were viewed for accuracy and manual cell reassignment was performed where necessary. Results were correlated to the ‘gold standard’ of manual microscopy for each WBC class, and sensitivity and specificity of blast identification were calculated.
Results: The AI digital differential was very strongly correlated to microscopy (r > .8) for most normal cell types and did not require any manual reassignment. The AI digital differential was less reliable for abnormal blood films (r = .50-.87), but could be greatly improved by manual assessment of digital images for most cell types (r > .95) with the exception of immature granulocytes (r = .62). For blast identification, initial AI digital differentials showed 96% sensitivity and 25% specificity, which was improved to 99% and 84%, respectively, after manual digital review.
Conclusions: The Techcyte platform allowed remote viewing and manual analysis of digitized slides that was comparable to microscopy. The AI software produced adequate WBC differentials for normal films and had high sensitivity for blast identification in malignant films.
Keywords: artificial intelligence; digital; haematologic; malignancy; morphology.
REFERENCES
- Kratz A, Lee S, Zini G, Riedl J, Hur M, Machin S. Digital morphology analyzers in hematology: ICSH review and recommendations. Int J Lab Hematol. 2019;41:437-447.
- Stossel TP. Blood: Principles and Practice of Hematology, vol. 1. Phildelphia: Lippincott Williams & Wilkinson; 2003.
- Stouten K, et al. Examination of peripheral blood smears; performance evaluation of a digital microscope system using a larger-scale leukocyte database. International Journal of Laboratory Hematology. 2015.
- Kratz A, et al. Performance evaluation of the CellaVision DM96 system WBC differentials by automated digital image analysis supported by an artificial neural network. Am J Clin Pathol. 2005;124(5):770-781.
- Yu H, et al. Evaluation of an Automated Digital Imaging System, Nextslide Digital Review Network, for Examination of Peripheral Blood Smears. Arch Pathol Lab Med. 2012;136(6):660-667
- Brugnara C. Automated Analyzers: State of the Art, An Issue of Clinica in Laboratory Medicine. E-Book: Elsevier Health Sciences; 2015.
- Kim HN, et al. Performance of automated digital cell imaging analyzer Sysmex DI-60. Clin Chem Lab Med. 2018;94-102.
- Merino A, Puigvi L, Boldu L, Alferez S, Rodellar J. Optimizing morphology through blood cell image analysis. Int J Lab Hematol. 2018;54-61.
- Wayne, PA. Validation, verification, and quality assurance of automated hematology analyzer; Approved standard-second edition. s.l. Clinical and Laboratory. Standards Institute (CLSI). 2010.
- Cornet E, Perol JP, Troussard X. Performance evaluation and relevance of the CellaAvision DM96 system in routine analysis and in patients with malignant hematological diseases. Int Jnl Lab Hem, 2008 536-542.
- Techcyte. Techcyte.[Online] 21 January 2020. techcyte.com/technology/.
- Sasada K, et al. Inter-observer variance and the need for standardization in the morphological classification of myelodysplastic syndrome. Leuk Res. 2018;69:54-59.
- Akoglu, H. User’s guide to correlation coefficients. Turkish J Emerg Med. 2018;18(3):91-93.
- Buttarello M, et al. Evaluation of four automated hematology analyzers. A comparative study of differential counts (imprecision and inaccuracy). Am J Clin Pathol. 1992;345-352.
- Greenway A, Monagle, eds. Abnormal haematology results in children, vol. 27. Melbourne: Aust Presc; 2004:64-66.
- Pantanowitz L, et al. Validating Whole Slide Imaging for Diagnostic Purposes in Pathology: Guideline from the College of American Pathologists Pathology and Laboratory Quality Center. Archives of Pathology & Laboratory Medicine. 2013;137(12):1710-1722.